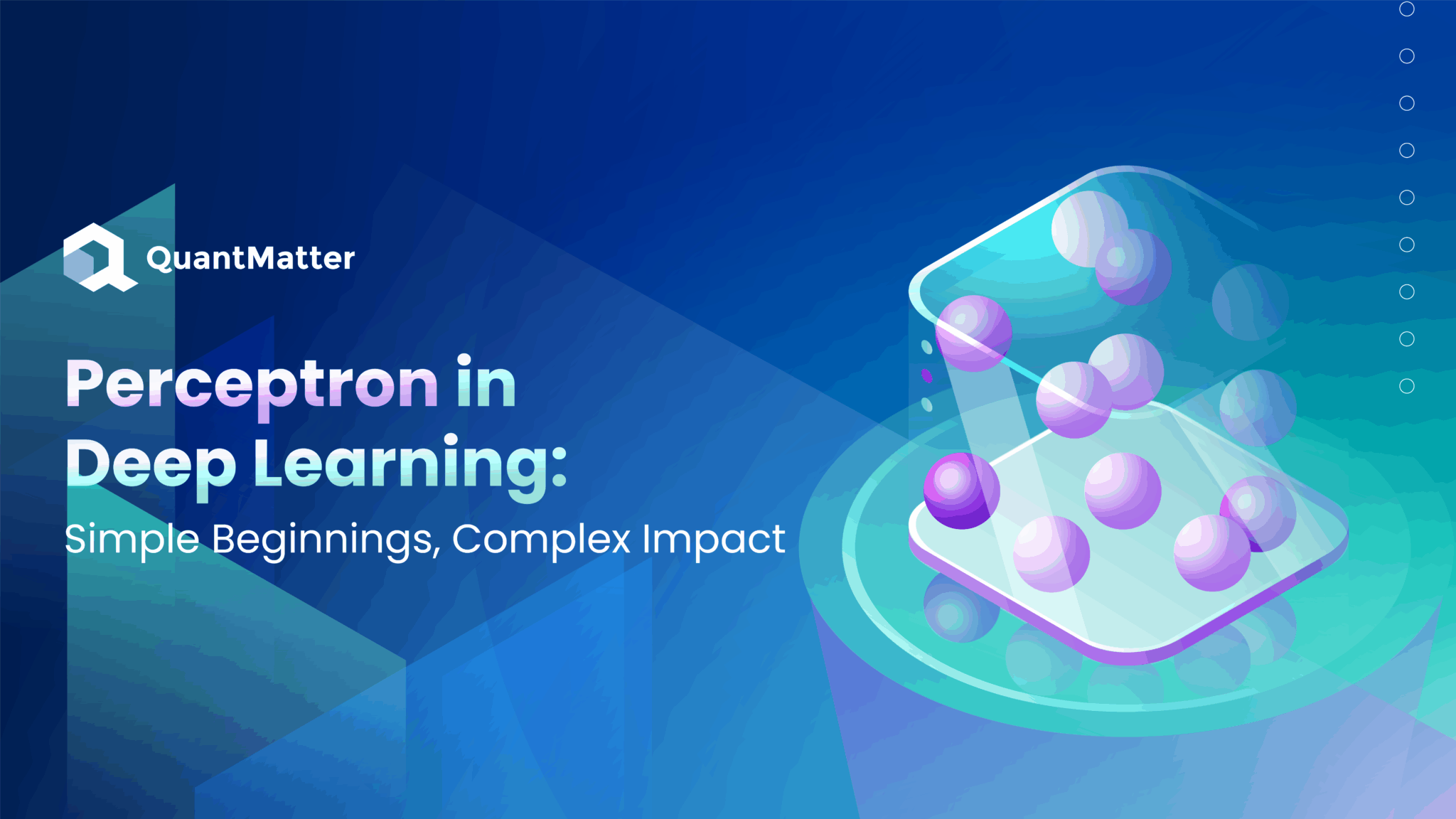
When people talk about deep learning, they often jump straight to powerful models like convolutional neural networks or transformers. But all of that complexity started with something much simpler: the perceptron. At its core, a perceptron is a basic type of artificial neuron—simple in design, yet groundbreaking in impact.
Back in the 1950s and 60s, the perceptron was introduced as a way to mimic the decision-making ability of a human brain. It couldn’t do much by today’s standards, but it proved something vital—that machines could learn. This small breakthrough laid the foundation for everything that came after.
Understanding the perceptron isn’t just about looking back at history. It’s about understanding the basic building blocks of today’s deep learning models. If you’re learning how deep learning works or trying to build a strong mental model of neural networks, you need to know the perceptron.
What Is a Perceptron?
A perceptron is a type of artificial neuron. Think of it as a machine that makes decisions based on input. It takes several inputs, multiplies each one by a weight, adds a bias, and then passes the result through an activation function. If the result crosses a certain threshold, it fires; otherwise, it doesn’t.
This might sound abstract, but here’s an example: imagine a perceptron that decides whether or not to approve a loan. It takes inputs like credit score, income, and debt. Each input has a weight, which represents its importance. The perceptron processes these inputs and outputs either a 1 (approve) or a 0 (deny). That’s it—simple logic based on weighted input.
The beauty of the perceptron lies in its simplicity. It doesn’t have hidden layers. It’s just a single layer with inputs and an output. This is why it’s also called a single-layer neural network.
The Birth of the Perceptron
The perceptron was first introduced by Frank Rosenblatt in 1958. He was trying to model how the human brain makes decisions using a machine. At the time, it was a revolutionary idea. Computers were seen as tools for calculations, not decision-making.
Rosenblatt’s original perceptron model was even built into hardware. It was called the Mark 1 Perceptron and was used for image recognition. It could recognize simple patterns and learn to improve its decisions over time. This was a big deal—it showed that machines could adjust based on data, something we now call “learning.”
But the early hype didn’t last. In 1969, researchers Marvin Minsky and Seymour Papert published a book that pointed out the perceptron’s major limitations. Specifically, they showed that a single-layer perceptron couldn’t solve problems like the XOR function—a simple logic problem where two inputs need to be compared in a way the perceptron couldn’t handle. Interest in neural networks faded for years.
Also Read: 10 Best Crypto Fund Management in Dubai – Updated List for 2025
How the Perceptron Works

At a glance, the perceptron might look like a simple math formula. But in reality, it’s a step-by-step process that mimics decision-making based on data. This process forms the base of all neural networks—even the most complex deep learning models rely on the same basic operations happening over and over again in layers.
Understanding how a perceptron works is key to understanding how machines learn. Let’s break it down.
Step 1: Input Features
The perceptron starts with input values, usually in numerical form. These values are often features from a dataset. For example, in a dataset predicting whether someone will buy a product, the inputs might include:
- Age
- Monthly income
- Time spent on the website
- Previous purchases
These inputs are passed into the perceptron as a vector. Each one provides a small piece of information that the model will weigh to make its final decision.
Step 2: Assigning Weights
Each input is multiplied by a weight, which tells the model how important that input is in making a decision. These weights are not fixed—they’re learned during training.
For example:
- If time spent on the website is more predictive than age, its weight will grow larger during training.
- If income has little impact, its weight might shrink or stay small.
At the beginning, these weights are set randomly. Over time, the model updates them to improve accuracy.
Step 3: Summation and Bias
Next, the perceptron adds up all the weighted inputs, along with a special number called a bias. The bias allows the model to shift the output up or down, helping it fit the data better.
This part of the process looks like this:
weighted_sum = (w1 * x1) + (w2 * x2) + … + (wn * xn) + bias
This sum is just a raw value. It still needs to be passed through a function to determine the final decision.
Step 4: Activation Function
The activation function decides whether the perceptron “fires” or not. In the original perceptron model, the step function was used:
- If the sum is above a certain threshold → output is 1 (positive class)
- If not → output is 0 (negative class)
This kind of binary output is useful for tasks like:
- Spam vs. not spam
- Approve vs. deny
- Yes vs. no
In more advanced models, other activation functions are used (like ReLU or sigmoid), but the core idea stays the same—transform the raw score into a decision.
Step 5: Learning Through Training
What really makes the perceptron powerful is its ability to learn from mistakes. After making a prediction, it checks whether it was right. If it was wrong, it adjusts the weights using a process called gradient descent.
This training process works like this:
- The model makes a prediction.
- It compares the prediction to the actual answer.
- It calculates the error (how far off it was).
- It adjusts the weights slightly to reduce the error.
- Repeat over many cycles (epochs) until the model improves.
This loop is the heart of machine learning. It’s how the model moves from random guesses to reliable predictions.
Summary Formula
Here’s the simplified version of the full perceptron operation:
output = activation(weight1 * input1 + weight2 * input2 + … + bias)
Each piece of that formula reflects a real part of the learning process—from the data you feed in to the decision the model makes.
Even though the perceptron is simple, it’s still relevant. Modern deep learning just stacks many of these units across layers and connects them in complex ways. The principles—input, weighted sum, activation, and learning—are all still there.
By learning how a single perceptron works, you’re learning the core logic behind today’s most advanced AI systems. Whether it’s recognizing faces, translating languages, or recommending videos, it all starts with this.
Limitations of the Perceptron
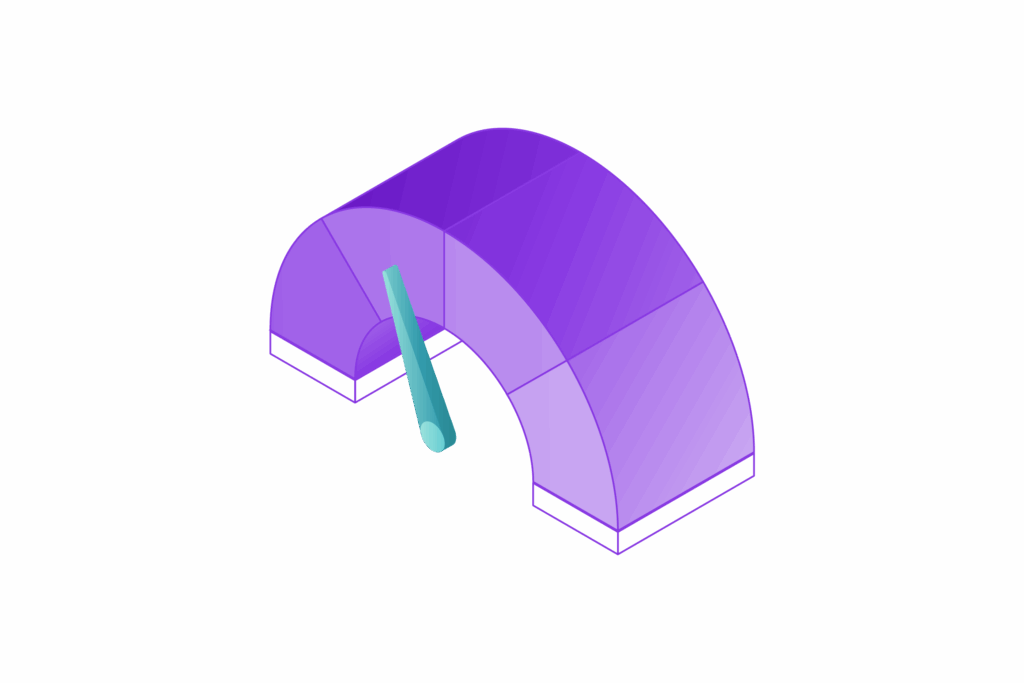
The single-layer perceptron has serious limitations. The most important one is that it can only solve linearly separable problems. That means the data has to be separable by a straight line (or a hyperplane in higher dimensions). This makes it ineffective for more complex tasks like image recognition, speech processing, or natural language understanding.
The XOR problem is a classic example. If you plot the XOR inputs and outputs on a graph, you’ll see that no straight line can separate the outputs. A single-layer perceptron just can’t solve it. This was the key criticism from Minsky and Papert, and it stalled progress in neural networks for almost two decades.
Another limitation is that the perceptron doesn’t provide probabilities. It gives binary decisions—yes or no, 0 or 1. This makes it less helpful for tasks where confidence scores matter, like ranking search results or classifying images.
Here’s a breakdown of key limitations:
Limitation | What It Means | Impact |
Only solves linearly separable data | Can’t separate complex patterns like XOR | Misses key relationships in real-world datasets |
No hidden layers | Lacks depth needed to model non-linear functions | Cannot solve multi-step problems or extract deep features |
Binary output only | Produces hard 0 or 1 decisions without confidence scores | Less useful for ranking, probabilistic tasks |
Poor performance on complex tasks | Not designed for image, text, or speech processing | Inadequate for most real-world applications |
While these limits make the perceptron too simple for many modern needs, they also highlight why later innovations—like multilayer networks—were so important.
From Perceptrons to Deep Learning
So if perceptrons are so limited, why are we still talking about them? Because they’re the foundation of everything that came after. Without understanding perceptrons, it’s hard to fully grasp how modern neural networks function. They may not be powerful on their own, but they introduced core ideas that are still central to deep learning today.
The turning point came with multi-layer perceptrons (MLPs). These models added one or more hidden layers between the input and output layers. With these layers, the network could learn non-linear relationships and handle more complex problems. The classic XOR problem? Solved. Image recognition, text classification, and speech processing? Now possible.
Key Advancements That Made Deep Learning Possible
Here’s how deep learning evolved from the simple perceptron:
- Hidden Layers: By stacking layers of neurons, MLPs could model more complex functions and patterns that a single-layer perceptron couldn’t.
- Non-linear Activation Functions: Functions like ReLU (Rectified Linear Unit), sigmoid, and tanh allowed networks to introduce non-linearity, which is essential for learning complicated mappings.
- Backpropagation: This algorithm made training multi-layer networks feasible. It works by calculating gradients and updating weights layer by layer, improving accuracy over time.
- Increased Computing Power: The rise of GPUs and cloud computing allowed deeper and wider networks to be trained on massive datasets.
- Regularization Techniques: Methods like dropout, batch normalization, and weight decay helped reduce overfitting and improved model performance.
Applications of Deep Learning Today
All of this led to the development of deep learning—the use of neural networks with many layers. These deep networks are made up of building blocks that are, in essence, glorified perceptrons. They still follow the same logic: take input, apply weights, sum, pass through an activation function, and repeat.
Today, deep learning powers technologies such as:
- Voice assistants – for real-time speech recognition and response
- Medical image analysis – identifying diseases from scans
- Recommendation systems – suggesting content on Netflix, YouTube, Spotify
- Language translation – converting text and speech across languages
- Self-driving cars – processing visual and sensor data to make decisions
Also Read: 7 Best Crypto Arbitrage to Check this 2025
Why the Perceptron Still Matters
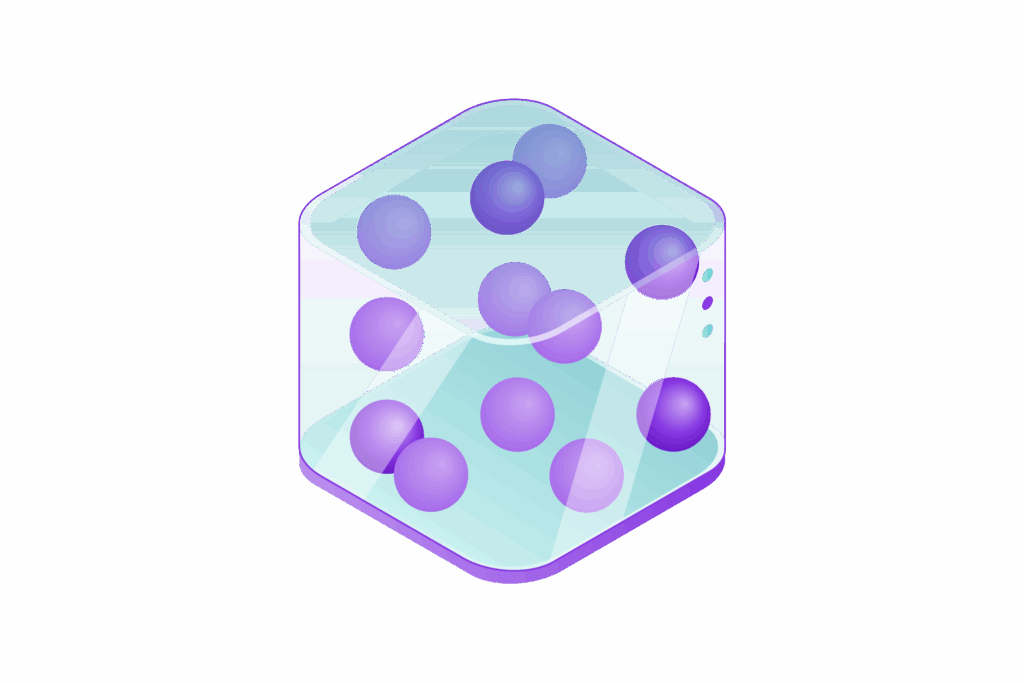
Even with all the breakthroughs in artificial intelligence, the perceptron still holds its ground. It may not be flashy or powerful on its own, but its value lies in clarity, simplicity, and influence. Whether you’re learning, building, or exploring, the perceptron offers a solid starting point.
Here’s why it continues to matter:
- Great for learning the basics: The perceptron is one of the clearest ways to understand how machines learn. You can see how inputs, weights, bias, and activation functions work together to produce output.
- Beginner-friendly: Many courses and tutorials start with building a perceptron from scratch. It helps make neural networks less of a black box by showing each step in action—how decisions are made and how learning happens.
- Useful in low-resource environments: In systems where deep learning is overkill or too resource-heavy (e.g., embedded devices, sensors), perceptron-based models can still deliver solid performance.
- Good enough for simple tasks: For basic classification or decision-making problems where the data is linearly separable, a perceptron can be accurate, fast, and easy to implement.
- Foundation of deep learning: Modern neural networks are built on the same core ideas—inputs, weights, sums, and activations. The perceptron introduces these concepts in their simplest form.
- Reminder of where AI started: In a field that changes quickly, the perceptron is a helpful reminder that big breakthroughs often start with small, simple ideas.
The perceptron’s simplicity is what makes it powerful. It teaches the essentials, works in the right scenarios, and connects us to the roots of deep learning. No matter how far the field goes, the perceptron will always have a place in the story.
Conclusion
The perceptron was a simple idea that sparked a massive shift in how we think about machines and learning. It couldn’t do much on its own, but it opened the door to something bigger. It proved that machines could learn from data—and that was enough to start a revolution.
From its early days in the 1950s to its role in shaping deep learning today, the perceptron has had an outsized impact. Modern models are more powerful, but they all build on the same core ideas. Every neuron in a deep network is, in essence, a distant cousin of the original perceptron.
If you’re learning about deep learning, don’t skip over the perceptron. Understanding it gives you a better grip on what’s happening under the hood of today’s complex models. And sometimes, the best way to understand something big is to start with something small.
Disclaimer: The information provided by Quant Matter in this article is intended for general informational purposes and does not reflect the company’s opinion. It is not intended as investment advice or a recommendation. Readers are strongly advised to conduct their own thorough research and consult with a qualified financial advisor before making any financial decisions.

I'm Carina, a passionate crypto trader, analyst, and enthusiast. With years of experience in the thrilling world of cryptocurrency, I have dedicated my time to understanding the complexities and trends of this ever-evolving industry.
Through my expertise, I strive to empower individuals with the knowledge and tools they need to navigate the exciting realm of digital assets. Whether you're a seasoned investor or a curious beginner, I'm here to share valuable insights, practical tips, and comprehensive analyses to help you make informed decisions in the crypto space.
- Carinahttps://quantmatter.com/author/carina/
- Carinahttps://quantmatter.com/author/carina/
- Carinahttps://quantmatter.com/author/carina/
- Carinahttps://quantmatter.com/author/carina/