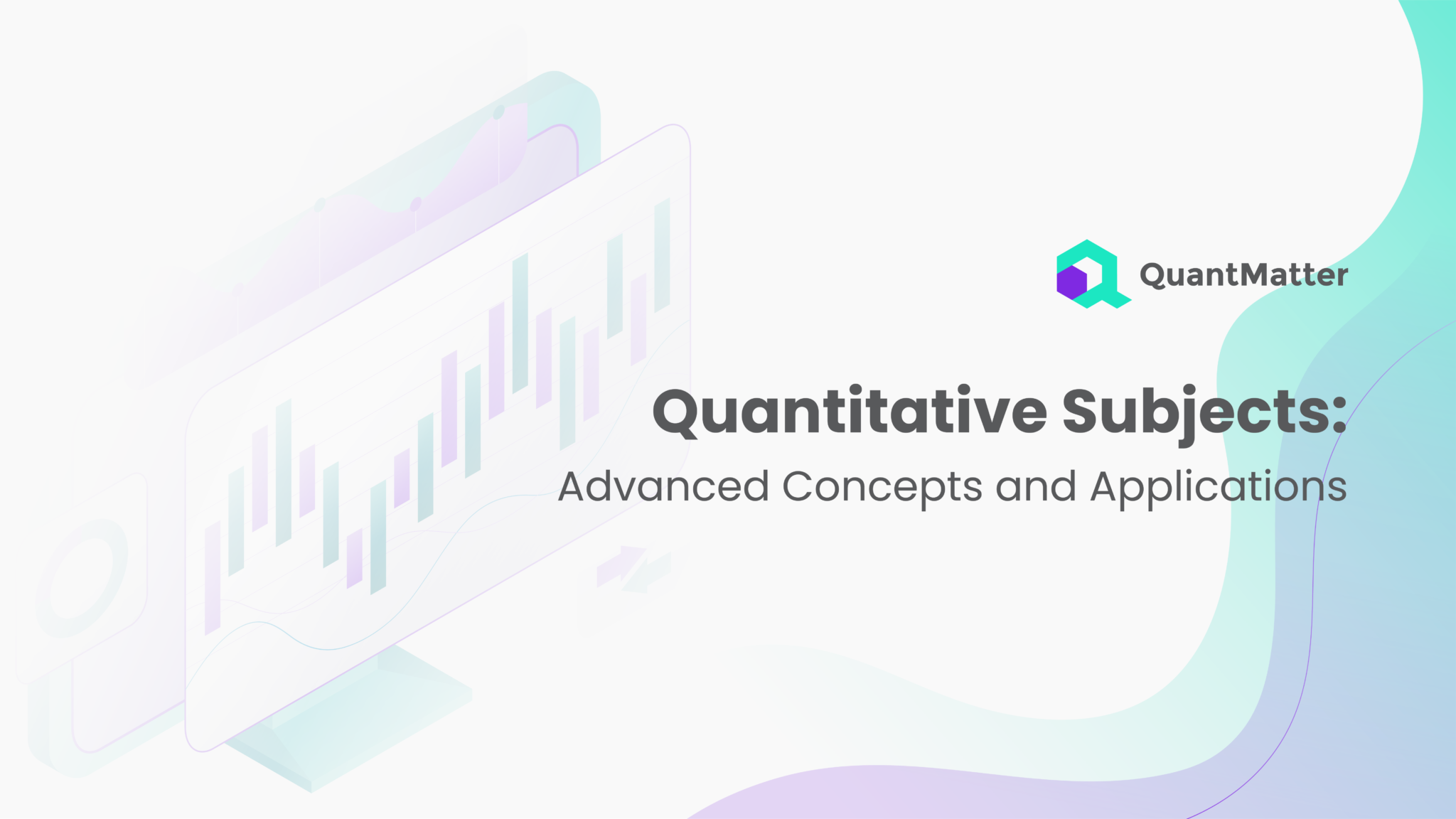
Quantitative subjects form the backbone of many scientific, economic, and technological advancements. From data analysis to financial modeling, understanding these subjects is crucial for making informed decisions and solving complex problems. In this article, we will delve into advanced concepts and applications of quantitative subjects, aiming to provide a comprehensive overview for students, professionals, and enthusiasts.
We will explore various quantitative methods and their real-world applications, offering insights into how these concepts are used in different fields. Whether you are looking to enhance your knowledge for academic purposes or to apply these techniques in your professional life, this guide will serve as a valuable resource.
By breaking down complex ideas into simpler terms and providing practical examples, we aim to make quantitative subjects more accessible and engaging. Let’s embark on this journey to deepen our understanding of quantitative concepts and their practical significance.
What Are Quantitative Subjects?
Quantitative subjects involve the study and application of mathematical, statistical, and computational techniques to analyze and interpret data. These subjects focus on quantifying relationships, patterns, and trends within data sets to make informed decisions and predictions. Common quantitative subjects include mathematics, statistics, data science, and quantitative finance.
The core principle of quantitative subjects is to use numerical data to derive meaningful insights. This approach is essential in various fields such as economics, engineering, natural sciences, and social sciences. By employing mathematical models and statistical tools, professionals can solve complex problems, optimize processes, and improve decision-making.
In academic settings, quantitative subjects are foundational for developing analytical skills. Students learn to apply theoretical concepts to real-world scenarios, preparing them for careers in research, industry, and beyond. The growing reliance on data-driven decision-making in today’s world underscores the importance of mastering quantitative subjects.
Advanced Statistical Methods
Advanced statistical methods are crucial for analyzing complex data sets and drawing accurate conclusions. These methods go beyond basic statistics, offering more sophisticated tools for data analysis.
Regression Analysis
Regression analysis is a powerful statistical technique used to understand the relationship between variables. It helps in predicting the value of a dependent variable based on one or more independent variables. Linear regression is the most common type, but there are other forms such as multiple regression, logistic regression, and polynomial regression. These techniques are widely used in economics, engineering, and social sciences for forecasting and determining causality.
Bayesian Statistics
Bayesian statistics provides a probabilistic approach to inference. It updates the probability of a hypothesis as more evidence becomes available. This method is particularly useful in situations where information is uncertain or incomplete. Bayesian methods are applied in various fields, including genetics, machine learning, and finance, for tasks such as parameter estimation, hypothesis testing, and decision making.
Also Read: Top 10 Stablecoins to Check in 2024 (Updated List)
Time Series Analysis
Time series analysis involves studying datasets that are collected over time. This method is essential for identifying trends, seasonal patterns, and cyclic behaviors in data. Techniques like autoregressive integrated moving average (ARIMA) and exponential smoothing are commonly used for time series forecasting. Applications include economic forecasting, weather prediction, and analyzing stock market trends.
Multivariate Analysis
Multivariate analysis examines more than two variables simultaneously to understand complex relationships. Techniques such as principal component analysis (PCA), factor analysis, and cluster analysis are used to reduce dimensionality, identify underlying structures, and group similar data points. This type of analysis is vital in fields like psychology, marketing, and bioinformatics, where multiple factors influence outcomes.
These advanced statistical methods provide a deeper understanding of data and enable more accurate predictions and decisions. They are fundamental tools for researchers and professionals working in data-intensive fields.
Applications in Data Science
Data science is an interdisciplinary field that uses scientific methods, processes, algorithms, and systems to extract knowledge and insights from structured and unstructured data. Advanced statistical methods play a critical role in data science, enabling the extraction of meaningful information and predictions from data.
Predictive Modeling
Predictive modeling involves using statistical techniques to create models that can predict future outcomes based on historical data. Techniques such as regression analysis, decision trees, and neural networks are commonly used. These models are essential in various industries, including finance, healthcare, and marketing, for tasks such as credit scoring, disease prediction, and customer segmentation.
Machine Learning
Machine learning is a subset of data science that focuses on building algorithms that allow computers to learn from and make decisions based on data. Techniques such as supervised learning, unsupervised learning, and reinforcement learning are used to build models that can identify patterns, make predictions, and improve over time. Applications include recommendation systems, image recognition, and natural language processing.
Big Data Analytics
Big data analytics involves analyzing large and complex data sets to uncover hidden patterns, correlations, and other insights. Tools such as Hadoop, Spark, and NoSQL databases are used to handle and process massive amounts of data efficiently. Big data analytics is crucial in fields like e-commerce, social media, and finance, where large volumes of data are generated continuously.
Data Visualization
Data visualization is the graphical representation of data to help stakeholders understand complex data sets and derive insights. Tools like Tableau, Power BI, and matplotlib enable data scientists to create interactive and visually appealing charts, graphs, and dashboards. Effective data visualization helps in making data-driven decisions by highlighting trends, outliers, and patterns.
Natural Language Processing (NLP)
Natural language processing is a branch of artificial intelligence that focuses on the interaction between computers and human language. NLP techniques are used to analyze and interpret text and speech data. Applications include sentiment analysis, language translation, and chatbots. NLP helps in extracting valuable insights from unstructured text data, making it an essential tool in data science.
The applications of data science are vast and varied, leveraging advanced statistical methods to solve real-world problems and drive innovation across industries.
Quantitative Finance Techniques
Quantitative finance applies mathematical models and computational techniques to analyze financial markets and securities. This field leverages advanced quantitative methods to make investment decisions, manage risks, and develop financial strategies.
Financial Modeling
Financial modeling involves creating detailed mathematical representations of a company’s financial performance, assets, or portfolios. These models are essential tools for decision-making in finance, helping analysts and investors predict future financial outcomes based on historical data and various assumptions. One widely used technique is Discounted Cash Flow (DCF) analysis, which estimates the value of an investment based on its expected future cash flows, discounted back to their present value. This method is critical for assessing the intrinsic value of companies and projects, guiding investment decisions.
Another key technique is the use of option pricing models, such as the Black-Scholes model, which helps in determining the fair price of options based on factors like the stock price, strike price, volatility, time to expiration, and the risk-free interest rate. Additionally, Monte Carlo simulations are employed to model the probability of different outcomes in processes that are inherently uncertain.
This method is particularly useful in assessing the risk and uncertainty in financial forecasts, investment appraisal, and portfolio management. By employing these techniques, financial modeling aids in valuing securities, assessing investment opportunities, and understanding the impact of various factors on financial performance, ultimately guiding strategic financial planning and risk management.
Risk Management
Risk management is a fundamental aspect of quantitative finance, focusing on identifying, assessing, and mitigating financial risks to protect the stability and profitability of financial institutions. One of the primary techniques used in risk management is Value at Risk (VaR), which measures the potential loss in value of a portfolio over a defined period for a given confidence interval. VaR provides a quantitative estimate of market risk and helps in determining the capital reserves necessary to cover potential losses.
Stress testing and scenario analysis are also crucial techniques in risk management. Stress testing involves evaluating the resilience of financial institutions under extreme but plausible adverse conditions, such as significant market downturns or economic crises. Scenario analysis, on the other hand, explores the impact of specific hypothetical events on a portfolio’s value, allowing for a more comprehensive understanding of potential risks. These methods are essential for regulatory compliance and ensuring financial institutions can withstand adverse market conditions. Quantitative risk management enables firms to make informed decisions, maintain stability, and comply with regulatory requirements by effectively measuring and mitigating potential financial risks.
Algorithmic Trading
Algorithmic trading, or algo trading, uses sophisticated computer algorithms to execute trades automatically based on predefined criteria. These algorithms analyze vast amounts of market data in real-time to identify trading opportunities, optimize trade execution, and minimize market impact. One popular technique in algorithmic trading is statistical arbitrage, which involves exploiting price discrepancies between correlated securities to generate profits. High-frequency trading (HFT), another common approach, uses powerful computers to execute a large number of orders at extremely high speeds, often within milliseconds.
Machine learning models are increasingly being employed in algorithmic trading to enhance the accuracy of predictions and adapt to changing market conditions. These models can analyze historical data, identify patterns, and learn from new data, continuously improving their trading strategies. By leveraging these quantitative models, algorithmic trading can enhance market efficiency and liquidity, reduce transaction costs, and provide a competitive edge in financial markets. The use of advanced algorithms and technology in trading has revolutionized the financial industry, leading to more efficient and sophisticated trading practices.
Portfolio Optimization
Portfolio optimization involves selecting the best combination of assets to achieve specific investment objectives while minimizing risk. This process is grounded in modern portfolio theory, which seeks to construct portfolios that maximize expected returns for a given level of risk. Mean-variance optimization, developed by Harry Markowitz, is a fundamental technique in portfolio optimization. It involves calculating the expected return and variance of each asset, as well as the covariances between assets, to identify the efficient frontier—a set of optimal portfolios offering the highest expected return for a given level of risk.
The Capital Asset Pricing Model (CAPM) is another essential tool used in portfolio optimization. CAPM describes the relationship between systematic risk and expected return, helping investors assess the risk-reward trade-off of individual assets. By incorporating these quantitative methods, investors can construct diversified portfolios that align with their risk tolerance and investment goals. Portfolio optimization not only helps in maximizing returns but also in managing risk, ensuring that investors achieve their financial objectives in a systematic and efficient manner.
Derivatives Pricing
Derivatives pricing involves valuing financial instruments whose value is derived from underlying assets, such as stocks, bonds, or commodities. Accurate pricing of derivatives is crucial for managing financial risks and developing effective hedging strategies. One of the most widely used techniques for pricing options is the Black-Scholes model, which calculates the fair price of options based on factors like the underlying asset price, strike price, volatility, time to expiration, and the risk-free interest rate. This model provides a theoretical estimate of option prices and has become a standard in the industry.
Binomial tree models are another important method for pricing derivatives. These models provide a flexible framework for valuing options by simulating different possible paths of the underlying asset price over time, allowing for the incorporation of various factors and assumptions. Monte Carlo simulations are also extensively used in derivatives pricing, particularly for complex derivatives with multiple sources of uncertainty. This technique involves running numerous simulations to model the probability distribution of the derivative’s value, providing a comprehensive understanding of potential outcomes. By employing these quantitative techniques, financial professionals can accurately price derivatives, manage financial risks, and develop effective hedging strategies to protect against adverse market movements.
Quantitative finance techniques are indispensable for modern financial markets, providing the tools and methods needed to analyze data, make informed decisions, and manage financial risks effectively.
Operations Research and Optimization
Operations research and optimization involve using mathematical models, statistical analyses, and algorithms to solve complex decision-making problems. These techniques are used to optimize processes, improve efficiency, and reduce costs across various industries.
Linear Programming
Linear programming is a mathematical technique used to optimize a linear objective function subject to linear constraints. This method is widely used in operations research for resource allocation, production planning, and scheduling problems. The Simplex method and interior-point methods are common algorithms used to solve linear programming problems.
Integer Programming
Integer programming is similar to linear programming but requires some or all variables to be integers. This technique is essential for solving problems where solutions must be whole numbers, such as in logistics, scheduling, and network design. Branch and bound, and cutting plane methods are common algorithms used in integer programming.
Network Optimization
Network optimization focuses on optimizing the flow of goods, information, or resources through a network. Techniques such as shortest path algorithms, maximum flow algorithms, and minimum spanning tree algorithms are used to solve problems related to transportation, telecommunications, and supply chain management. These techniques help in minimizing costs and improving the efficiency of network operations.
Queuing Theory
Queuing theory studies the behavior of waiting lines or queues. This theory is used to analyze and optimize systems where resources are limited and must be shared among multiple users, such as in customer service, manufacturing, and telecommunications. Queuing models help in understanding system performance, predicting wait times, and improving service efficiency.
Also Read: What is Intrinsic Value? Understanding the Core Concept
Simulation
Simulation involves creating a computer model of a real-world system to study its behavior under different scenarios. This technique is used in operations research to analyze complex systems that are difficult to model analytically. Monte Carlo simulation and discrete-event simulation are common methods used to simulate and optimize processes in manufacturing, healthcare, and logistics.
Game Theory
Game theory analyzes competitive situations where the outcome depends on the actions of multiple decision-makers. This technique is used in economics, business strategy, and military planning to understand strategic interactions and optimize decision-making. Nash equilibrium, zero-sum games, and cooperative games are key concepts in game theory.
Operations research and optimization techniques provide powerful tools for improving decision-making and efficiency in various fields. They help organizations to optimize their processes, allocate resources effectively, and enhance overall performance.
Conclusion
Quantitative subjects encompass a wide range of disciplines that use mathematical and statistical methods to analyze data and solve complex problems. From advanced statistical methods to applications in data science, quantitative finance, and operations research, these subjects are integral to many fields. By employing sophisticated techniques such as regression analysis, machine learning, financial modeling, and optimization algorithms, professionals can derive valuable insights and make informed decisions.
The importance of quantitative methods is evident in their diverse applications. In data science, they enable predictive modeling, big data analytics, and natural language processing, driving innovation and efficiency across industries. In finance, quantitative techniques are essential for risk management, portfolio optimization, and algorithmic trading, ensuring stability and profitability in financial markets. Operations research and optimization techniques improve decision-making and resource allocation, enhancing operational efficiency in various sectors.
As the world becomes increasingly data-driven, the demand for expertise in quantitative subjects continues to grow. Mastering these techniques is crucial for students and professionals seeking to excel in research, industry, and beyond. Whether it’s through developing new algorithms, analyzing financial markets, or optimizing complex systems, the applications of quantitative methods are vast and impactful. By understanding and applying these advanced concepts, individuals can contribute to advancements in technology, improve organizational performance, and make better decisions in their professional and personal lives.
Disclaimer: The information provided by Quant Matter in this article is intended for general informational purposes and does not reflect the company’s opinion. It is not intended as investment advice or a recommendation. Readers are strongly advised to conduct their own thorough research and consult with a qualified financial advisor before making any financial decisions.
Joshua Soriano
As an author, I bring clarity to the complex intersections of technology and finance. My focus is on unraveling the complexities of using data science and machine learning in the cryptocurrency market, aiming to make the principles of quantitative trading understandable for everyone. Through my writing, I invite readers to explore how cutting-edge technology can be applied to make informed decisions in the fast-paced world of crypto trading, simplifying advanced concepts into engaging and accessible narratives.
-
Joshua Soriano#molongui-disabled-link
-
Joshua Soriano#molongui-disabled-link
-
Joshua Soriano#molongui-disabled-link
-
Joshua Soriano#molongui-disabled-link