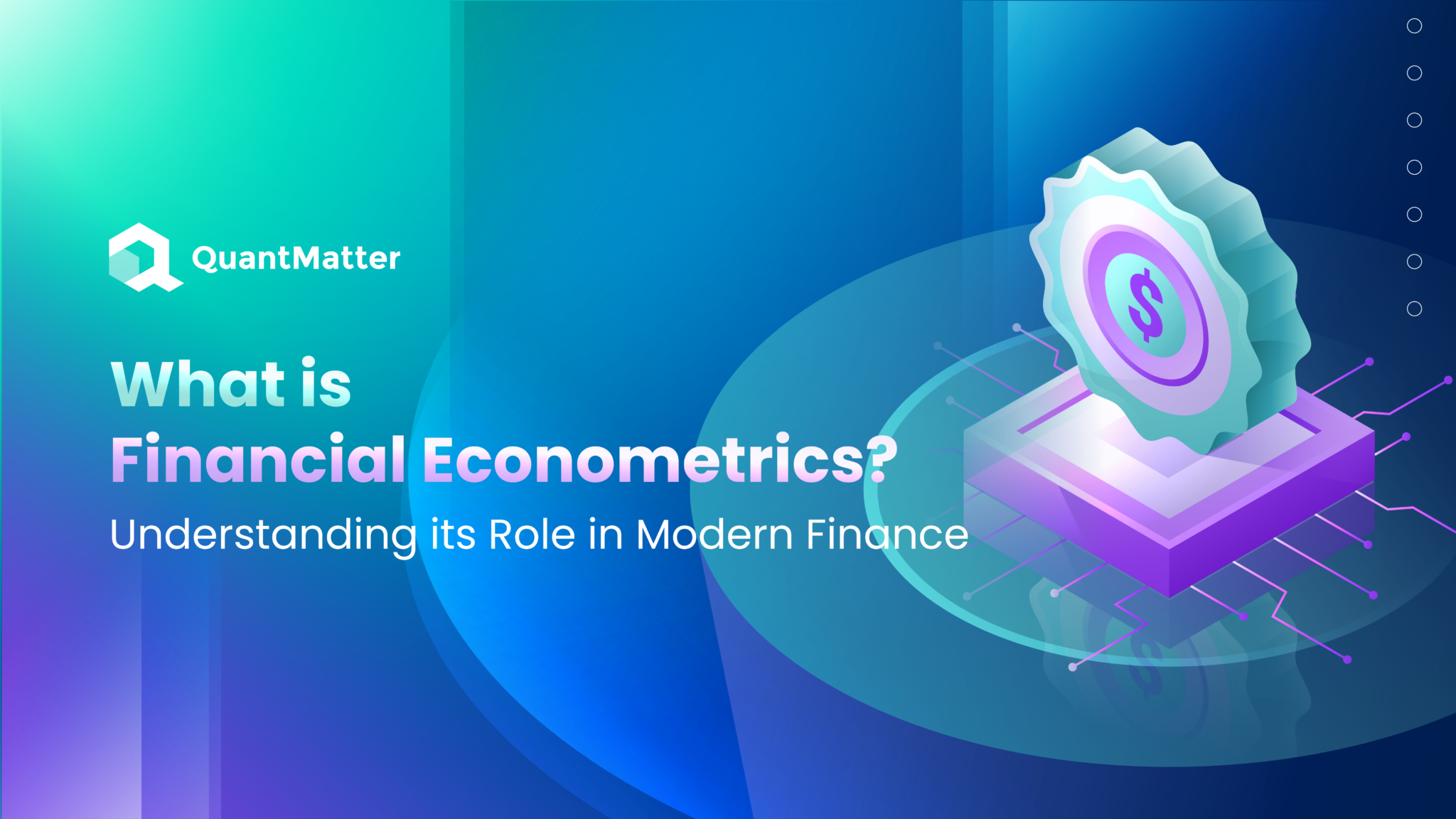
Financial markets generate vast amounts of data every day. Investors, analysts, and policymakers need tools to make sense of this data, predict trends, and manage risks. Financial econometrics helps them do just that by applying statistical methods to financial data.
At its core, financial econometrics bridges the gap between theoretical finance and real-world data. It allows economists to test financial theories, build forecasting models, and analyze market behavior. Without financial econometrics, understanding stock prices, interest rates, and economic trends would be much harder.
This article explores what financial econometrics is, why it matters, the key techniques used, its applications, and the challenges it faces. By the end, you will have a solid grasp of this essential field in modern finance.
What Is Financial Econometrics?
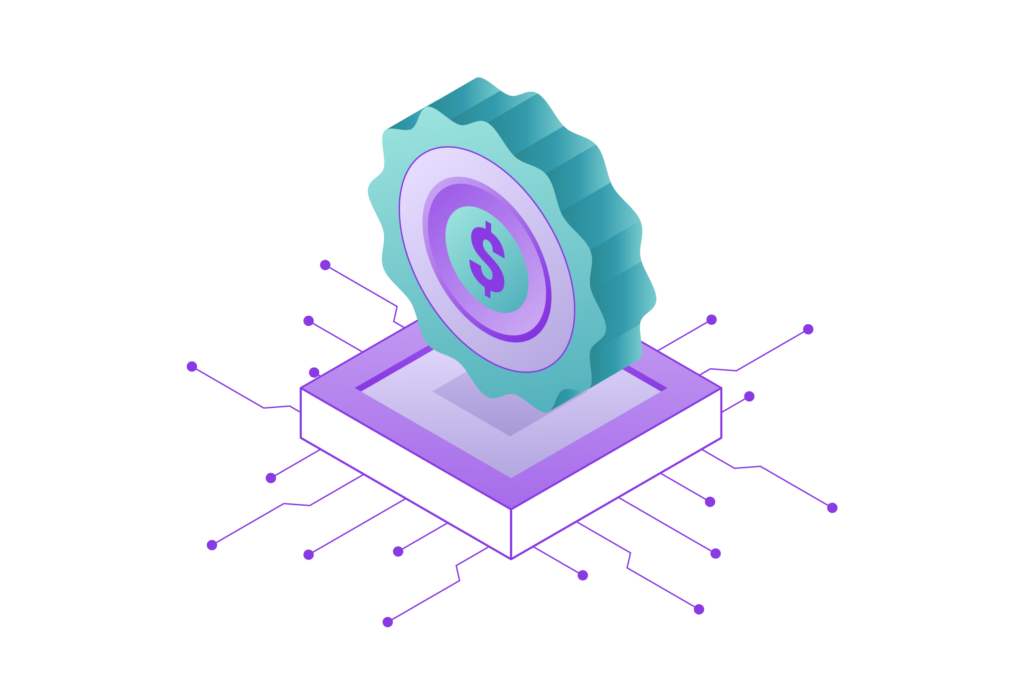
Financial econometrics is the application of statistical and mathematical techniques to financial data. It helps analyze financial markets, test theories, and make predictions about future trends. This field combines finance, statistics, and programming to solve real-world financial problems.
Econometrics itself is a broader field that applies statistical methods to economic data. Financial econometrics is a specialized branch focused on financial markets, investment decisions, risk management, and pricing models. It plays a crucial role in understanding market efficiency, asset pricing, and portfolio optimization.
Financial econometrics uses real data rather than just theoretical models. Analysts and researchers rely on historical stock prices, interest rates, bond yields, and other financial indicators to test economic theories. These models help investors and policymakers make informed decisions.
Why Financial Econometrics Matters
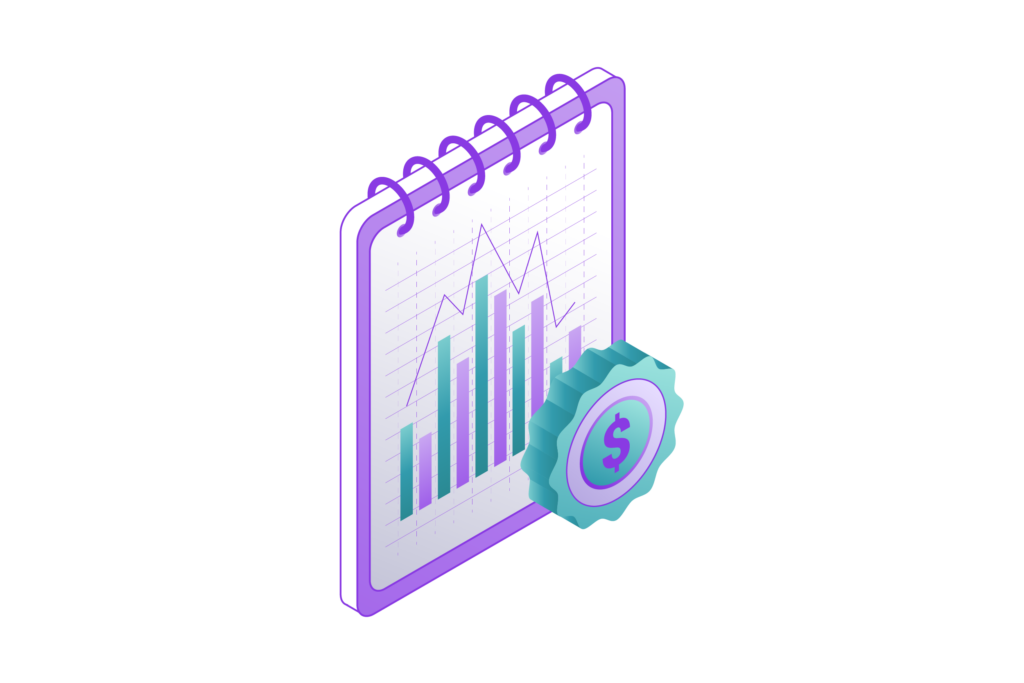
Financial markets are unpredictable, and making the right investment decisions requires a deep understanding of risk, return, and market behavior. Financial econometrics provides tools to analyze these factors, helping businesses, investors, and governments make better financial decisions.
One of its key contributions is in risk management. Banks, hedge funds, and asset managers use financial econometrics to measure risk exposure and prevent financial crises. By analyzing past market behavior, they can build models that predict possible losses and prepare for uncertainties.
Another critical area is market efficiency. Financial econometrics helps test whether markets are truly efficient, meaning all available information is reflected in asset prices. If markets are inefficient, there could be opportunities for investors to gain higher-than-average returns.
Also Read: Why a Masters in Finance Won’t Make You a Quant Trader?
Key Techniques in Financial Econometrics
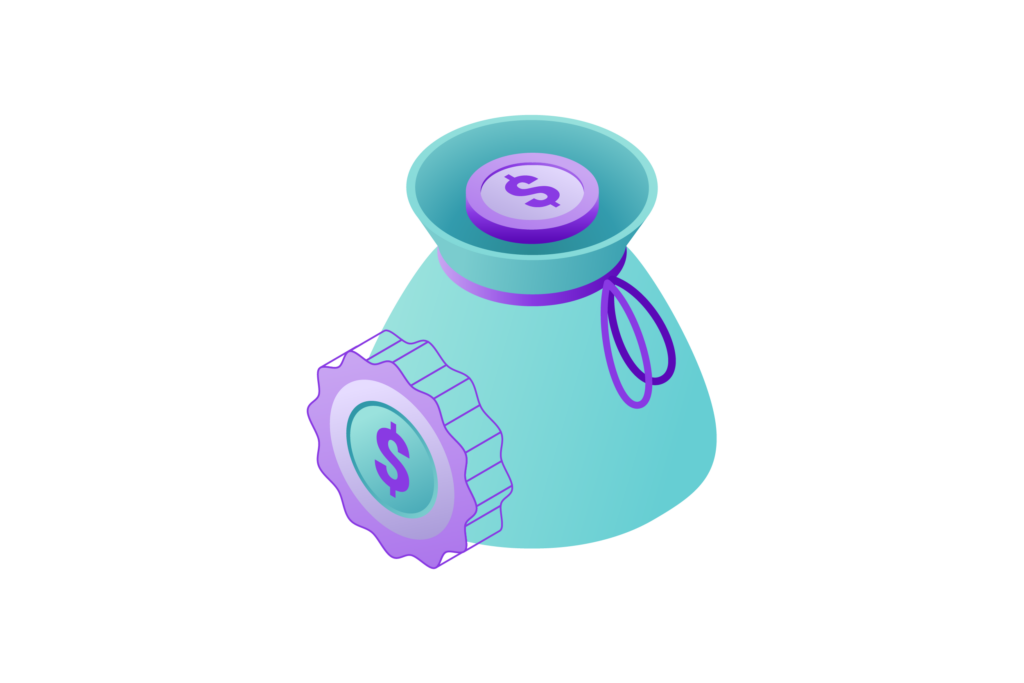
Financial econometrics relies on a variety of statistical techniques. Some of the most common ones include:
1. Time Series Analysis
Financial data is often collected over time, making time series analysis essential. This technique helps analyze trends, detect patterns, and forecast future movements in stock prices, exchange rates, and interest rates.
Common time series models include:
- Autoregressive (AR) models: These use past values to predict future values.
- Moving Average (MA) models: These smooth out fluctuations by averaging past data points.
- Autoregressive Moving Average (ARMA) models: A combination of AR and MA models for better predictions.
- Autoregressive Integrated Moving Average (ARIMA) models: These account for trends and seasonal effects.
2. Regression Analysis
Regression analysis helps determine relationships between variables. For example, analysts might study how interest rates affect stock prices.
Two common types of regression used in financial econometrics are:
- Ordinary Least Squares (OLS): Estimates the relationship between independent and dependent variables.
- Logistic Regression: Used for predicting binary outcomes, such as whether a stock price will go up or down.
3. Volatility Modeling
Understanding and predicting volatility is crucial in finance. Investors need to assess how much risk they are taking on.
Popular models for measuring volatility include:
- Generalized Autoregressive Conditional Heteroskedasticity (GARCH) models: Used to forecast changing market volatility.
- Exponential Weighted Moving Average (EWMA): Places more weight on recent data points.
4. Machine Learning in Financial Econometrics
With the rise of big data, machine learning techniques are becoming more common in financial econometrics. Algorithms like neural networks, decision trees, and support vector machines are used to identify patterns, detect anomalies, and predict market movements.
Applications of Financial Econometrics
Financial econometrics is a crucial tool in finance, helping professionals make data-driven decisions. It is widely used in various areas, including asset pricing, risk management, portfolio optimization, algorithmic trading, and economic forecasting. Below, we explore these applications in greater detail.
1. Asset Pricing
One of the core questions in finance is how assets, such as stocks, bonds, and derivatives, are priced. Financial econometrics helps in developing and testing asset pricing models, which explain how different factors influence asset returns.
Key Models in Asset Pricing:
- Capital Asset Pricing Model (CAPM): This model explains the relationship between expected return and risk, where investors are compensated for taking on additional risk. Financial econometrics is used to test whether CAPM holds true in real-world markets.
- Fama-French Three-Factor Model: An extension of CAPM, this model adds size and value factors to better explain stock returns. Financial econometricians analyze large datasets to determine if small-cap and value stocks outperform the market.
- Arbitrage Pricing Theory (APT): This model accounts for multiple risk factors affecting asset returns. Financial econometrics helps identify which macroeconomic variables influence stock prices.
By applying statistical methods, analysts can determine whether markets are correctly pricing assets or if there are mispricing opportunities that investors can exploit.
2. Risk Management
Risk is inherent in financial markets, and financial econometrics provides the tools to measure, analyze, and manage risk effectively. Banks, investment firms, and insurance companies rely on econometric models to assess their exposure to different types of risks, such as market risk, credit risk, and liquidity risk.
Common Risk Management Techniques:
- Value-at-Risk (VaR): This model estimates the potential loss in an investment portfolio over a specific time frame with a given level of confidence. Financial econometrics is used to calculate VaR based on historical data and probability distributions.
- Conditional Value-at-Risk (CVaR): Also known as Expected Shortfall (ES), this model improves on VaR by considering extreme losses beyond the VaR threshold.
- Stress Testing: Financial institutions use econometric techniques to simulate adverse economic scenarios, such as a financial crisis or a sharp rise in interest rates, to assess their ability to withstand shocks.
- Credit Risk Modeling: Banks use financial econometrics to estimate the likelihood of borrowers defaulting on their loans. Popular models include logistic regression and machine learning techniques like decision trees and neural networks.
Effective risk management is essential for financial stability, and financial econometrics plays a key role in identifying, quantifying, and mitigating financial risks.
3. Portfolio Optimization
Investors seek to maximize returns while minimizing risk, and financial econometrics helps identify the optimal mix of assets in a portfolio. Portfolio optimization models use historical return data and risk metrics to find the best investment strategy.
Key Portfolio Optimization Models:
- Markowitz’s Modern Portfolio Theory (MPT): This theory suggests that investors should diversify their portfolios to achieve the highest possible return for a given level of risk. Financial econometrics is used to estimate expected returns, standard deviations, and correlations between assets.
- Mean-Variance Optimization (MVO): This method calculates the most efficient frontier, which represents the best risk-return combinations available in the market.
- Black-Litterman Model: This model enhances traditional portfolio optimization by incorporating investor views and subjective probabilities.
Financial econometrics helps asset managers and retail investors make informed decisions about asset allocation, balancing risks and rewards to achieve their financial goals.
4. Algorithmic Trading
Financial markets have become increasingly automated, with algorithmic trading accounting for a significant portion of daily trading volume. Financial econometrics is at the heart of algorithmic trading strategies, where data-driven models help traders make high-frequency and low-latency trades.
Key Components of Algorithmic Trading:
- Quantitative Analysis: Financial econometrics helps traders analyze historical price patterns, correlations, and seasonality to develop trading strategies.
- Machine Learning and AI: Techniques such as neural networks and support vector machines are used to identify profitable patterns in large financial datasets.
- Statistical Arbitrage: Traders use econometric models to find mispriced assets and execute trades that exploit market inefficiencies.
- Market Microstructure Analysis: This involves studying bid-ask spreads, order flow, and liquidity to improve trade execution and minimize slippage.
With the rise of big data and high-speed computing, financial econometrics is becoming even more critical in designing and optimizing trading algorithms.
5. Economic Forecasting
Governments, central banks, and financial institutions rely on economic forecasting to make policy and investment decisions. Financial econometrics helps in building predictive models for key economic indicators such as GDP growth, inflation, unemployment, and interest rates.
Key Forecasting Techniques:
- Time Series Models: Autoregressive Integrated Moving Average (ARIMA) and Vector Autoregression (VAR) models help predict future economic trends based on past data.
- Macroeconomic Models: Large-scale econometric models incorporate multiple variables to forecast economic growth and inflation trends.
- Event Studies: Financial econometricians analyze the impact of policy changes, financial crises, and global events on economic performance.
Accurate economic forecasting helps policymakers design effective monetary and fiscal policies, while investors use these predictions to adjust their portfolio strategies.
6. Option Pricing and Derivatives Valuation
Financial derivatives, such as options and futures, require precise pricing models to determine their fair value. Financial econometrics is used extensively in derivatives markets to develop and validate pricing models.
Popular Option Pricing Models:
- Black-Scholes Model: This widely used model calculates the fair price of European options using factors like stock price, strike price, volatility, and time to expiration.
- Binomial Tree Model: This model provides a flexible framework for pricing American options, which can be exercised before expiration.
- Monte Carlo Simulations: These simulations use random sampling techniques to estimate the value of complex derivatives.
Accurate pricing of derivatives is essential for traders, market makers, and risk managers who rely on these financial instruments for hedging and speculation.
7. Behavioral Finance and Market Sentiment Analysis
Financial econometrics is increasingly being used to study investor behavior and market sentiment. Traditional financial theories assume that investors act rationally, but behavioral finance suggests that psychological biases influence financial decisions.
Applications in Behavioral Finance:
- Sentiment Analysis: By analyzing news articles, social media, and earnings reports, financial econometricians can gauge investor sentiment and its impact on stock prices.
- Herding Behavior Analysis: Financial econometrics helps detect periods when investors follow the crowd, leading to asset bubbles or market crashes.
- Market Anomalies: Analysts use econometric models to identify patterns like the January effect, momentum trading, and mean reversion strategies.
Understanding behavioral biases allows investors and regulators to anticipate market movements and prevent irrational investment decisions.
Financial econometrics plays a crucial role in modern finance, helping professionals make data-driven decisions across various domains. From asset pricing and risk management to portfolio optimization and algorithmic trading, its applications are vast and growing.
As financial markets evolve and data becomes more complex, financial econometrics will continue to develop new models and techniques. Whether predicting economic trends, managing risk, or designing trading strategies, this field remains essential for academics, policymakers, and industry professionals alike.
Also Read: 12 Best Fastest Blockchain Platforms to Know in 2025
Challenges in Financial Econometrics
Challenges in Financial Econometrics
Financial econometrics has transformed the way financial professionals analyze markets, assess risk, and forecast economic trends. However, despite its many benefits, this field faces several challenges that impact the accuracy and reliability of its models. Issues such as data limitations, market unpredictability, and computational constraints make it difficult to develop consistently effective financial models. Understanding these challenges is crucial for improving financial econometric methods and ensuring that decision-makers can rely on data-driven insights.
The table below summarizes some of the major challenges in financial econometrics:
Challenge | Description |
Data Limitations | Financial data may be noisy, incomplete, or manipulated, leading to unreliable predictions. |
Changing Market Conditions | Financial markets evolve rapidly, requiring constant model updates to stay relevant. |
Overfitting | Complex models may fit historical data too well but fail in predicting future trends. |
Computational Complexity | Advanced econometric models require significant computing power and expertise. |
1. Data Limitations
One of the biggest challenges in financial econometrics is dealing with poor-quality data. Financial datasets can be noisy, meaning they contain irrelevant or misleading information that affects model accuracy. Additionally, data is often incomplete due to missing historical records or reporting errors. Fraudulent manipulation, such as companies inflating earnings reports, can also distort the accuracy of econometric models.
To overcome these issues, financial analysts use data-cleaning techniques, such as removing outliers, filling missing values, and cross-validating multiple sources. However, despite these efforts, unreliable data remains a significant obstacle in financial econometrics.
2. Changing Market Conditions
Financial markets are highly dynamic, and models built on historical data may not always reflect current realities. Factors such as economic crises, policy changes, and global events can disrupt existing trends and make previously reliable models ineffective.
For example, the 2008 financial crisis invalidated many risk models that had been considered robust. Similarly, events like the COVID-19 pandemic showed how unexpected shocks can lead to massive market volatility, making traditional econometric models less useful.
To address this challenge, financial econometricians continuously refine their models by incorporating real-time data, using adaptive algorithms, and employing stress testing to anticipate worst-case scenarios.
3. Overfitting
Overfitting occurs when a model becomes too complex and captures patterns in past data that do not generalize to future events. This problem is especially common when financial analysts use excessive variables to create predictive models. While a model may seem highly accurate based on past data, it can fail when applied to new situations.
A common example is hedge funds using sophisticated machine learning models that perform exceptionally well in backtesting but fail in live trading conditions. To mitigate overfitting, financial econometricians use simpler models, cross-validation techniques, and out-of-sample testing to ensure that models remain useful over time.
4. Computational Complexity
As financial econometrics incorporates machine learning and big data techniques, the demand for computational resources has increased. Many econometric models involve complex calculations that require significant processing power and technical expertise.
For example, Monte Carlo simulations, deep learning algorithms, and high-frequency trading models require large-scale computations that can only be handled by specialized hardware. This poses challenges for small firms and independent analysts who may not have access to advanced computing infrastructure.
To tackle this issue, many financial institutions use cloud computing and parallel processing techniques to enhance model efficiency. Additionally, new software tools are being developed to make complex econometric modeling more accessible to a wider audience.
Despite its challenges, financial econometrics remains an essential field in modern finance. By addressing data limitations, adapting to changing markets, avoiding overfitting, and managing computational complexity, financial professionals can improve the accuracy and reliability of their models.
As technology advances, new solutions such as artificial intelligence and improved data collection methods will help mitigate these challenges. While financial econometrics will always face obstacles, continuous innovation ensures that it remains a powerful tool for understanding financial markets and making data-driven decisions.
Conclusion
Financial econometrics plays a vital role in understanding and managing financial markets. By applying statistical methods to financial data, it helps investors, businesses, and policymakers make informed decisions.
The field continues to evolve with the rise of big data and machine learning. As technology improves, financial econometrics will become even more powerful in predicting market trends and managing risks.
While challenges like data limitations and model overfitting exist, ongoing research and innovation help overcome these issues. Financial econometrics remains an essential tool in modern finance, shaping investment strategies and economic policies worldwide.
Disclaimer: The information provided by Quant Matter in this article is intended for general informational purposes and does not reflect the company’s opinion. It is not intended as investment advice or a recommendation. Readers are strongly advised to conduct their own thorough research and consult with a qualified financial advisor before making any financial decisions.
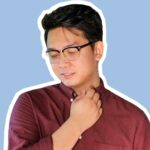
Joshua Soriano
As an author, I bring clarity to the complex intersections of technology and finance. My focus is on unraveling the complexities of using data science and machine learning in the cryptocurrency market, aiming to make the principles of quantitative trading understandable for everyone. Through my writing, I invite readers to explore how cutting-edge technology can be applied to make informed decisions in the fast-paced world of crypto trading, simplifying advanced concepts into engaging and accessible narratives.
- Joshua Soriano#molongui-disabled-link
- Joshua Soriano#molongui-disabled-link
- Joshua Soriano#molongui-disabled-link
- Joshua Soriano#molongui-disabled-link