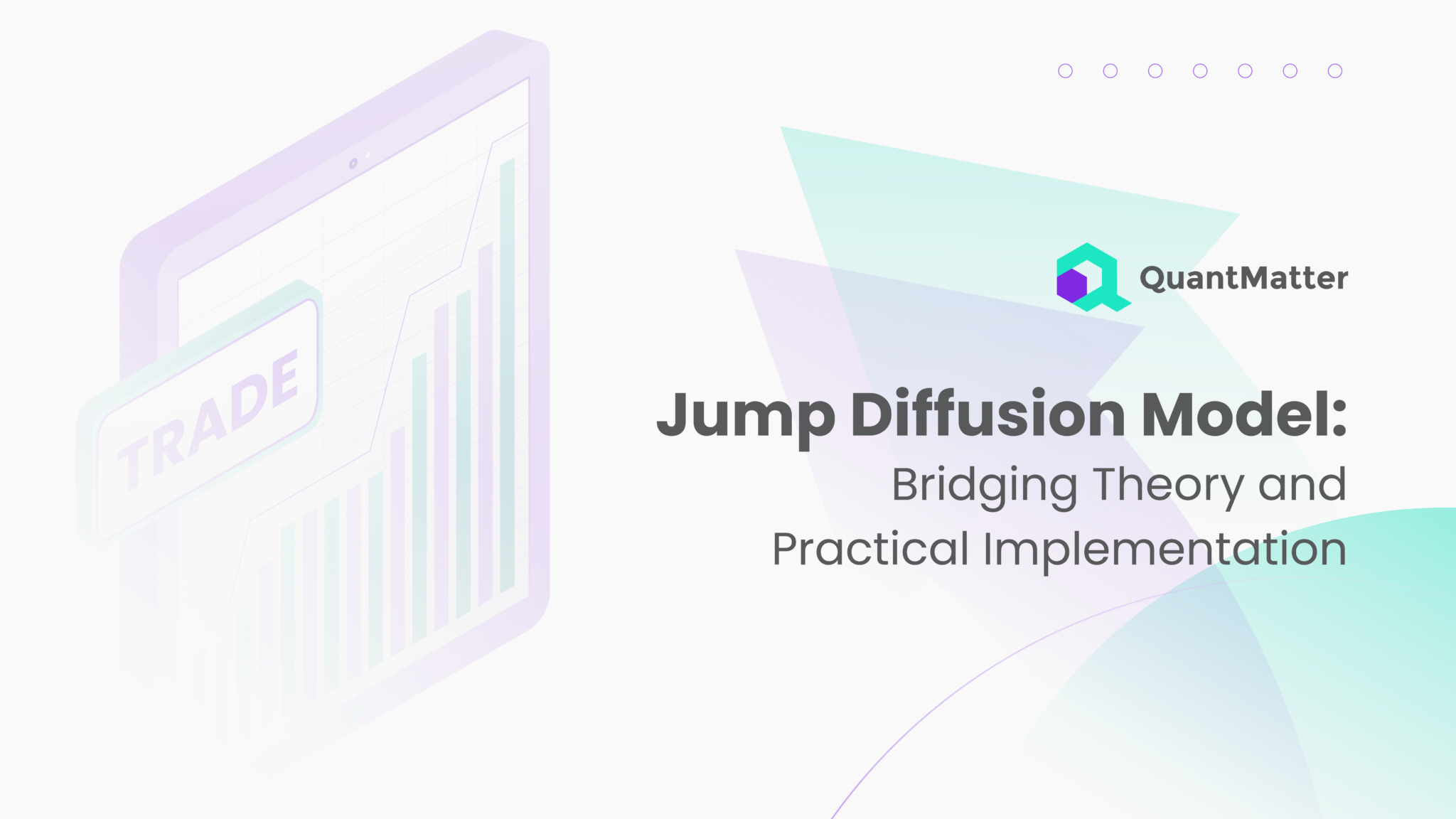
In the world of financial modeling, accurately predicting market behavior is crucial for making informed decisions. The jump diffusion model, a sophisticated approach blending continuous price changes with sudden jumps, provides a robust framework for capturing the complexities of financial markets. This model enhances the classic Black-Scholes framework by incorporating sudden, unpredictable events that can significantly impact asset prices.
Understanding the jump diffusion model is essential for finance professionals and students seeking to grasp the dynamics of market movements. By bridging the gap between theory and practical implementation, this model offers a comprehensive tool for analyzing and forecasting market trends. Whether dealing with stock prices, interest rates, or commodity markets, the jump diffusion model provides a nuanced view that traditional models often overlook.
In this article, we will delve into the theoretical foundations of the jump diffusion model, explore its practical applications in financial modeling, and examine its advantages and limitations. Additionally, we will present case studies and real-world examples to illustrate how this model is applied in various financial scenarios. By the end, you will have a solid understanding of the jump diffusion model and its significance in the realm of financial analysis.
What is Jump Diffusion Model?
The jump diffusion model is a financial model that accounts for both the continuous movement and sudden jumps in asset prices. It extends the traditional Black-Scholes model, which assumes that prices follow a continuous path influenced by a normal distribution. The jump diffusion model, however, recognizes that real-world markets experience sudden and significant changes, such as economic events, market crashes, or unexpected news.
At its core, the jump diffusion model combines two components: a continuous diffusion process and a jump process. The continuous diffusion process captures the regular, day-to-day price movements based on historical volatility. The jump process, on the other hand, introduces sudden changes in prices, modeled using a Poisson distribution. This dual approach allows for a more accurate representation of asset price dynamics.
Also Read: What is Quant Finance? Meaning and Elements
The parameters of the jump diffusion model include the jump intensity, which measures the frequency of jumps, and the jump size, which determines the magnitude of these jumps. By calibrating these parameters to historical data, the model can be tailored to reflect the unique characteristics of different financial instruments. This flexibility makes the jump diffusion model a powerful tool for analysts and traders seeking to navigate the uncertainties of financial markets.
Theoretical Foundations of the Jump Diffusion Model
The theoretical basis of the jump diffusion model was established by Robert Merton in 1976, who extended the Black-Scholes model to account for jumps in asset prices. Merton realized that assuming continuous price movements was inadequate for capturing the actual behavior of financial markets, which often experience sudden and unpredictable events.
In the jump diffusion model, asset prices are assumed to follow a path that includes both regular, continuous movements and occasional, discrete jumps. The continuous part reflects the usual day-to-day fluctuations, influenced by factors such as supply and demand, interest rates, and general market sentiment. This is similar to the assumptions made in the Black-Scholes model.
The jumps, however, are modeled separately and are based on a Poisson process, which provides a statistical framework for predicting the frequency and size of sudden price changes. The frequency of jumps is determined by a parameter known as the jump intensity, while the size of the jumps is typically assumed to follow a certain distribution, such as a normal distribution.
By combining these two elements—continuous diffusion and discrete jumps—the jump diffusion model offers a more comprehensive framework for understanding asset price dynamics. It can be tailored to different financial instruments by adjusting the parameters to match historical data, thereby improving the accuracy of forecasts and valuations.
This theoretical framework is crucial for various financial applications, including the pricing of options and other derivatives, risk management, and portfolio optimization. It allows financial analysts and traders to incorporate the likelihood of sudden market shifts into their models, leading to more robust and realistic predictions.
Practical Applications in Financial Modeling
The jump diffusion model has a wide range of practical applications in financial modeling, making it an essential tool for professionals in the field. Its ability to account for sudden and significant market movements provides a more realistic framework for various financial tasks.
Option Pricing
One of the primary applications of the jump diffusion model is in option pricing. Traditional models like Black-Scholes assume continuous price movements, often underestimating the risk of sudden price jumps. The jump diffusion model, however, incorporates these jumps, offering more accurate pricing of options, especially for those that are sensitive to large market movements, such as out-of-the-money options. This leads to better hedging strategies and risk management practices.
Risk Management
In risk management, understanding and predicting potential market shocks is crucial. The jump diffusion model allows risk managers to simulate scenarios that include sudden jumps, providing a more comprehensive view of potential risks. This helps in designing strategies to mitigate the impact of adverse market movements, such as financial crises or major economic announcements. By incorporating jumps, financial institutions can better estimate value-at-risk (VaR) and expected shortfall, leading to more effective risk management policies.
Portfolio Optimization
For portfolio managers, the jump diffusion model aids in optimizing asset allocation by considering the probability and impact of sudden price changes. Traditional models may overlook these jumps, potentially leading to suboptimal asset allocation. By using the jump diffusion model, portfolio managers can better diversify their portfolios, balancing the trade-off between risk and return more effectively. This results in portfolios that are more resilient to market shocks.
Credit Risk Analysis
In credit risk analysis, the jump diffusion model can be used to assess the likelihood of default events, which often occur suddenly. By modeling the jump intensity and size, analysts can estimate the probability of default and potential losses more accurately. This is particularly useful for pricing credit derivatives and managing credit portfolios.
Commodity Markets
In commodity markets, prices are often influenced by sudden geopolitical events, natural disasters, and other unexpected factors. The jump diffusion model captures these sudden price changes, providing a more accurate framework for pricing commodity futures and options. Traders and analysts can use this model to develop better trading strategies and manage risks associated with commodity price volatility.
Advantages and Limitations of the Jump Diffusion Model
The jump diffusion model offers several advantages that make it a valuable tool in financial modeling. However, it also has limitations that need to be considered when applying it in practice.
Advantages
- Realistic Market Representation: The jump diffusion model provides a more realistic representation of financial markets by accounting for both continuous price movements and sudden jumps. This dual approach captures the complexity of market behavior better than models that assume only continuous changes.
- Enhanced Risk Management: By incorporating the possibility of sudden jumps, the model allows for more accurate risk assessment and management. Financial institutions can use the model to simulate extreme scenarios and develop strategies to mitigate potential losses from sudden market shifts.
- Improved Option Pricing: Traditional models like Black-Scholes often underestimate the value of options due to their assumption of continuous price paths. The jump diffusion model offers more accurate option pricing, especially for options that are sensitive to large price movements, leading to better hedging strategies.
- Versatility: The jump diffusion model can be applied to a wide range of financial instruments and markets, including equities, commodities, and credit derivatives. Its flexibility makes it a useful tool for various financial applications, from pricing derivatives to optimizing portfolios.
Limitations
- Complexity: The jump diffusion model is more complex than traditional models, requiring a deeper understanding of stochastic processes and advanced mathematical techniques. This complexity can make it challenging to implement and calibrate, especially for those without a strong background in quantitative finance.
- Parameter Estimation: Estimating the parameters of the jump diffusion model, such as jump intensity and jump size distribution, can be difficult. Accurate estimation requires extensive historical data and sophisticated statistical methods. Inaccurate parameter estimation can lead to misleading results.
- Computational Intensity: The model’s complexity often translates to higher computational requirements. Simulating scenarios and calibrating the model can be computationally intensive, requiring significant processing power and time, which may be a constraint for some users.
- Assumptions and Limitations: Like all models, the jump diffusion model is based on certain assumptions, such as the distribution of jump sizes and the independence of jumps. These assumptions may not always hold true in real-world markets, potentially leading to inaccuracies in predictions.
Despite its limitations, the jump diffusion model remains a powerful tool for financial analysts and traders. By acknowledging its constraints and carefully calibrating its parameters, users can leverage its strengths to gain valuable insights into market behavior and enhance their decision-making processes.
Case Studies and Real-World Examples
The jump diffusion model has been employed in various real-world scenarios to improve financial modeling and decision-making. Here, we explore a few case studies and examples that highlight its practical applications.
Case Study 1: Option Pricing during Market Crises
During the 2008 financial crisis, many traditional option pricing models failed to account for the extreme volatility and sudden market crashes. Financial institutions that incorporated the jump diffusion model were better equipped to price options accurately. By considering the likelihood of sudden jumps, these institutions could adjust their pricing strategies to reflect the heightened risk environment. This approach not only provided more realistic valuations but also helped in developing effective hedging strategies against extreme market movements.
Case Study 2: Risk Management in Commodity Markets
A major energy trading firm adopted the jump diffusion model to manage risk in the oil market, which is highly susceptible to geopolitical events and natural disasters. By modeling the jump intensity and magnitude, the firm was able to simulate scenarios involving sudden price spikes and drops. This enhanced their risk management framework, allowing them to prepare for and mitigate the financial impact of unexpected events. As a result, the firm was able to maintain stable operations and protect its financial health during periods of extreme volatility.
Also Read: How to Become a Quant : Step by Step
Case Study 3: Portfolio Optimization in Emerging Markets
An investment fund focused on emerging markets used the jump diffusion model to optimize its portfolio. Emerging markets are known for their high volatility and susceptibility to political and economic shocks. By incorporating jumps into their asset pricing models, the fund managers could better assess the risk-return trade-off of different assets. This led to a more resilient portfolio that could withstand sudden market disruptions. The fund’s performance improved significantly, with reduced losses during market downturns and enhanced returns during stable periods.
Case Study 4: Credit Risk Analysis in Corporate Bond Markets
A leading credit rating agency implemented the jump diffusion model to analyze the risk of corporate bond defaults. Traditional models often underestimated the probability of sudden defaults, leading to inaccurate risk assessments. By modeling the jumps, the agency could better estimate the likelihood and impact of sudden credit events. This improved the accuracy of their ratings and provided investors with more reliable information for making investment decisions. The agency’s enhanced analytical capabilities were recognized by clients, leading to increased trust and business growth.
Case Study 5: Derivative Pricing for Financial Institutions
A global investment bank utilized the jump diffusion model to price complex financial derivatives. These instruments, often exposed to sudden market shifts, require precise pricing to manage risk effectively. The bank’s quantitative analysts used the model to incorporate the probability of jumps in their pricing algorithms. This allowed them to offer more competitive and accurate prices for derivatives, improving their market position and profitability. The model’s application also helped in better managing the bank’s risk exposure, ensuring long-term financial stability.
These case studies demonstrate the versatility and effectiveness of the jump diffusion model in various financial contexts. By providing a more comprehensive understanding of market dynamics, the model enables better pricing, risk management, and investment strategies.
Conclusion
The jump diffusion model represents a significant advancement in financial modeling by integrating both continuous price movements and sudden jumps. This comprehensive approach captures the complexities of financial markets more accurately than traditional models, making it essential for finance professionals and students. By incorporating the likelihood of sudden market shifts, the model enhances decision-making processes in pricing options, managing risk, and optimizing investment portfolios.
Despite its advantages, the jump diffusion model’s complexity and computational intensity present challenges. It requires a solid understanding of advanced mathematical and statistical techniques, and accurate parameter estimation can be difficult. However, the model’s benefits, particularly in high-risk applications, outweigh these challenges. Real-world applications, from option pricing during market crises to risk management in commodity markets, underscore its practical value.
The jump diffusion model bridges the gap between theoretical finance and practical implementation, providing a powerful tool for navigating the uncertainties of financial markets. By embracing this model, financial professionals can enhance their analytical capabilities and improve their strategic decisions. The jump diffusion model stands as a testament to the ongoing evolution of financial modeling, adapting to meet the demands of a dynamic market environment.
Disclaimer: The information provided by Quant Matter in this article is intended for general informational purposes and does not reflect the company’s opinion. It is not intended as investment advice or a recommendation. Readers are strongly advised to conduct their own thorough research and consult with a qualified financial advisor before making any financial decisions.
Joshua Soriano
As an author, I bring clarity to the complex intersections of technology and finance. My focus is on unraveling the complexities of using data science and machine learning in the cryptocurrency market, aiming to make the principles of quantitative trading understandable for everyone. Through my writing, I invite readers to explore how cutting-edge technology can be applied to make informed decisions in the fast-paced world of crypto trading, simplifying advanced concepts into engaging and accessible narratives.
-
Joshua Soriano#molongui-disabled-link
-
Joshua Soriano#molongui-disabled-link
-
Joshua Soriano#molongui-disabled-link
-
Joshua Soriano#molongui-disabled-link